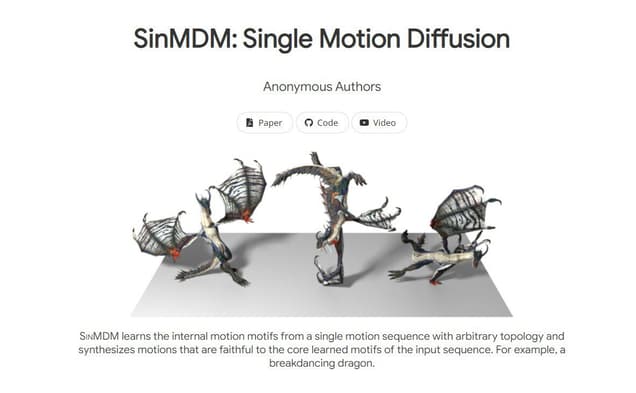


What is SinMDM?
SinMDM is a highly effective mobile device management (MDM) solution that businesses can rely on to efficiently manage their mobile devices. With a wide range of features, SinMDM ensures that devices remain secure and compliant with industry standards. This solution enables businesses to easily configure and remotely manage their mobile devices, making it suitable for businesses of any size.
By utilizing SinMDM, businesses can effortlessly set up and oversee user profiles, implement security policies, monitor device usage and performance, and have control over app installations. Additionally, users will benefit from an intuitive dashboard that simplifies the management of multiple devices. SinMDM also provides comprehensive support for various mobile platforms, including iOS, Android, Windows, and Blackberry.
Trusted by businesses worldwide, SinMDM stands out for its swift setup process and user-friendly features. It strikes the perfect balance between security and usability, empowering businesses to quickly deploy and manage their mobile devices without compromising the safety of their valuable data.
Information
- Price
- Contact for Pricing
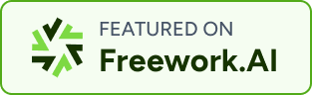
Freework.ai Spotlight
Display Your Achievement: Get Our Custom-Made Badge to Highlight Your Success on Your Website and Attract More Visitors to Your Solution.
Website traffic
- Monthly visits540
- Avg visit duration00:03:53
- Bounce rate76.80%
- Unique users--
- Total pages views719
Access Top 5 countries
Traffic source
SinMDM FQA
- What is the goal of SinMDM?
- What is the architecture of SinMDM?
- What applications can SinMDM be used for?
- How does SinMDM handle complex and non-trivial skeletons?
- Can SinMDM generate a crowd performing similar motions?
SinMDM Use Cases
SinMDM learns the internal motion motifs from a single motion sequence with arbitrary topology and synthesizes motions that are faithful to the core learned motifs of the input sequence. For example, a breakdancing dragon.
SinMDM is designed to be a lightweight architecture, which avoids overfitting by using a shallow network with local attention layers that narrow the receptive field and encourage motion diversity.
SinMDM can be applied in various contexts, including spatial and temporal in-betweening, motion expansion, style transfer, and crowd animation.
Our results show that SinMDM outperforms existing methods both in quality and time-space efficiency.
During inference, SinMDM can generate a crowd performing a variety of similar motions, such as hopping ostriches, jaguars, or breakdancing dragons.
The network can generate diverse results for the same inputs, and the completed motion naturally matches the given parts even when they are very different.
Motion composition can be done spatially by using selected joints as input and generating the rest.
In the case of style transfer, the model is trained on the style motion, and the content motion is adjusted to match the style motion's motifs.
Our network can synthesize variable length motions and generate very long animations with no additional training.