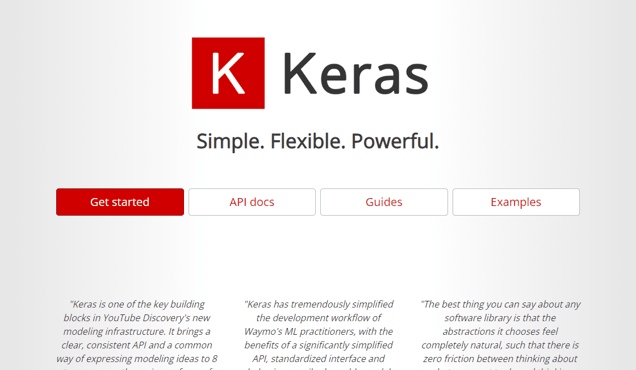


What is Keras Tool?
Keras is an open-source library that simplifies the process of building and deploying deep learning models for machine learning. It provides a high-level API that allows developers to easily create, train, and validate their models. With Keras, users can construct complex models with minimal code, enabling quick prototyping and the development of powerful solutions. The library supports various types of neural networks, including convolutional, recurrent, and generative models. Keras aims to make deep learning more accessible by offering an intuitive API for developers to prototype and build solutions effortlessly. It also offers advanced features for building more complex models. Additionally, Keras is optimized for both CPU and GPU computing, enabling users to leverage the speed and scalability of both architectures effectively.
Information
- Price
- Contact for Pricing
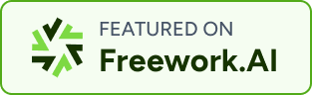
Freework.ai Spotlight
Display Your Achievement: Get Our Custom-Made Badge to Highlight Your Success on Your Website and Attract More Visitors to Your Solution.
Website traffic
- Monthly visits556.58K
- Avg visit duration00:02:56
- Bounce rate55.63%
- Unique users--
- Total pages views1.43M
Access Top 5 countries
Traffic source
Keras Tool FQA
- What are the benefits of using Keras?
- What do users like the most about Keras?
- What is Keras's purpose?
- Can Keras scale to large clusters of GPUs or TPUs?
- What is the role of Keras in the TensorFlow ecosystem?
Keras Tool Use Cases
Keras Tool is used by YouTube Discovery's new modeling infrastructure to bring a clear, consistent API and a common way of expressing modeling ideas to 8 teams across YouTube recommendations.
Keras Tool simplifies the development workflow of Waymo's ML practitioners by providing a significantly simplified API, standardized interface and behaviors, easily shareable model building components, and highly improved debuggability.
Keras Tool allows for intuitive and streamlined development, prototyping, research, and deployment of deep learning models. It offers an effective knowledge transfer between scientists and enables effective code comprehension and stylistic coding.
Keras Tool provides easy customizability for academics, out-of-the-box performant models and pipelines for industry use, and readable, modular code for students. It simplifies the iteration over experiments without worrying about low-level details.
Keras Tool is the perfect abstraction layer to build and operationalize Deep Learning models. It allows for the development and deployment of models for some of the largest companies in the world.
Keras Tool makes it easy to transition from research to production by training models, converting them to TF Lite, and deploying them to mobile and edge devices.
Keras Tool is user-friendly, easy to learn, and easy to use. It allows for rapid prototyping and deployment of models across various platforms.
Keras Tool is designed for human beings, offering consistent and simple APIs, reducing cognitive load, and providing clear and actionable error messages. It prioritizes great documentation and developer guides.
Keras Tool gives developers an unfair advantage in shipping Machine Learning-powered apps. It focuses on debugging speed, code elegance and conciseness, maintainability, and deployability.
Keras Tool is built on top of the TensorFlow platform, making it an industry-strength framework that can scale to large clusters of GPUs or an entire TPU pod.
Keras Tool can be deployed anywhere using the full deployment capabilities of the TensorFlow platform. It can be exported to JavaScript to run in the browser, TF Lite to run on iOS, Android, and embedded devices, or served as a web API.
Keras Tool is a central part of the tightly-connected TensorFlow ecosystem, covering every step of the machine learning workflow and offering solutions for data management, hyperparameter training, and deployment.
Keras Tool is used by scientific organizations such as CERN, NASA, NIH, and more. It provides low-level flexibility for implementing research ideas and high-level convenience features for speeding up experimentation cycles.